As language models grow beyond the capabilities of AI, the shift to non-Transformer structures becomes novel and appealing. In this article, we discuss ZAMBA2 7B, a language model that does not use a transformer and which was developed by Zyer, and which has figured in debates within the AI community. It is confidence that this model will be a success, a 7 billion parameter high performing model. ZAMBA2 was built to have high efficacy with computing power usage and total cost. However, does the implementation of ZAMBA2 in practical tasks work to its high grand design? How does ZAMBA2 7B performs on sophisticated tasks involving programming, reasoning and concepts for that matter?
NOTE: This is an official Research Paper by “CLOXLABS”
— Understanding the Rise of Non-Transformer Models
Transformers have been behind the groundbreaking of transformation of models GTP-3 and BERT in large languages. Yet, there are non-transformer models which are likely to address some of the issues associated with using transformer models. For instance, faster computational times, less power requirements, and a simpler structural design. ZAMBA2 uses as a base Mamba, a new architectural design that Zyer expects is a potential solution for some of the problems brought forth by a transformer, especially for users seeking efficiency within certain operational contexts.
All Ads on this website are served by GOOGLE
— Methodology: Evaluating ZAMBA2 7B’s Capabilities
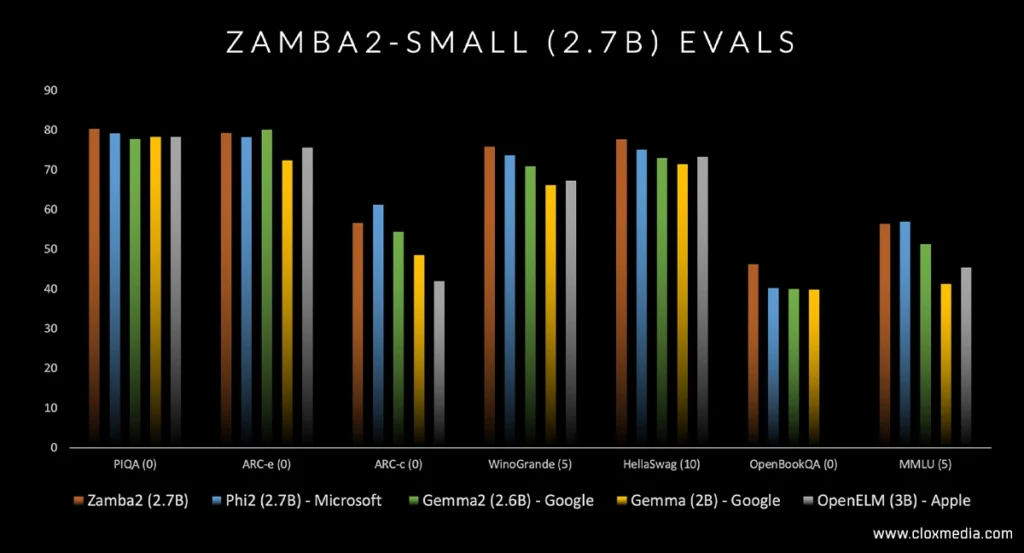
In order to be able to evaluate ZAMBA 2 7B fairly, the researchers constructed a fair range of tests covering various aspects of language. The model was placed for evaluation together with other models based on Transformer architectures Mistral 7B, Gemma 7B and LLaMA 3 8b. The main emphasis was on:
- Coding Tasks: Measuring its capacity to create code for a basic Tetris game or Snake-game.
- Logical Reasoning: If there is a change in performance on multi-step logical tasks.
- Basic Language Processing: Checking the accuracy of word count and letter count in language.
- Mathematical and Ethical Queries: Determine how well it is able to answer arithmetic problems and what it states it has done to get around any particular ethical problems.
Such a methodology guaranteed that a balanced evaluation was made taking into account these benchmark high claims against the real capabilities of Non-Transformer models.
— RESULTs: A HUGE… mess!
It has been interesting to find out how ZAMBA2 struggles and what areas are stronger with respect to these evaluations. Let’s break down its performance across the key metrics where they were evaluated.
Coding Tasks: This was an area where ZAMBA2 showed clear limitations. It displayed rather severe issues when it was tasked to come up with the complete working code segments for games such as Tetris and Snake, often resulting in either nonexistent or erratic returns. This was however a deviation from claims of it being robust. Mamba architecture has been said to be receptive to simplifications for some tasks, but evidently complex code seems to be beyond its reach as transformer architectures appear to be best suited for such hierarchical structures.
Logical Reasoning: If one thought that the reasoning was sound, then ZAMBA2 refined that notion when it came to accomplishing logical tasks. In instances which involved answering a stepwise approach with multiple such steps, the model repeated the mistake of providing the wrong answer on multiple occasions. One possibility is that the design of the Mamba model limits its reasoning ability as it’s incapable of recalling previous mechanisms stepwise designed which is one of the key features of Transformers.
Basic Language Processing: Even a simple pronunciation like counting letters, ZAMBA2 showed a certain degree of incapability of performing, the model was still very sharpened up with ZAMBA2. This variability indicates that while benchmarks might yield reasonable numbers, the actual application does not perform similarly because the model performs basic low-level text functions poorly.
Mathematical and Ethical Reasoning: Oddly, ZAMBA2 performed rather well on simple calculations and questions pertaining to ethics with a reasonable level of accuracy and language. Nonetheless, this improvement by itself is inadequate to place it as a serious option for general language processing errands considering the earlier failures of the model.
However, Allow me to explain what I realised on Real-World Testing Diverges from Benchmark Results?
The reality of ZAMBA2 was however his strength in the above metrics since in reality his tasks targeting the national championships were of extreme disappointment. This mismatch between apparent benchmarks and ideal scenario Use Case prompts thoughts on model building and evaluation. Some factors that possibly could aid in understanding these differences include:
- Architecture Constraints: There is no Mamba structure where presence of attention mechanism in Transformers helps to keep in memory contextual information helping to perform tasks involving cross sentence or cross code block relationships understanding.
- Benchmark Limitations: There could be a chance of non-Transformer models working well under benchmarks due to their limitations focusing on a single metric or too many that do not yield results in real life use.
- Data Quality and Scope: Perhaps the training data of ZAMBA2 along with its evaluation mechanisms need to be improved so that the model can perform well for tasks involving two or more steps of reasoning or text generation tasks requiring fidelity.

In conclusion: the role of ZAMBA2 7B in the evolution of the NLP field Despite the gaps that ZAMBA2 has in comparison to some of its more popular competitors it fully confirms the existence of non Transformer models worldwide. These non Transformer models, in this case ZAMBA2 displays somewhat promise in efficiency and ease of computation. This understanding of the ZAMBA 2 model should remind the reader that True AI systems, which have been touted by so many, remain a goal, and that benchmarks are a very important singular aspect of what has to be taken into account when assessing the real life efficacy of AI. Sequentially, the AI industry and science itself will have to grow both in terms of methodology and aesthetics: Transformer models will evolve, and non-Transformer models will also evolve, exhibiting their own resistance and opportunities.
All Ads on this website are served by GOOGLE
About the Author:
Amir Ghaffary is a visionary entrepreneur and AI expert at the forefront of digital media innovation. The visionary founder and CEO of CLOXMEDIA, a worldwide media network established in 2020 that focuses on cutting-edge AI technology. His education background is robust in computer science and digital art, as well as generations in the studying of AI and media psychology. This allowed him to combine technical skills with creativity in the field of digital media.
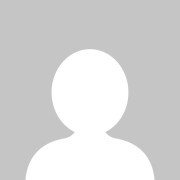
All Ads on this website are served by GOOGLE