It seems this week is all about successors to the transformer architecture. Among the significant announcements, SakanaAI has unveiled a groundbreaking approach to large language models (LLMs) in their new paper, “Transformer Squared: Self-Adaptive LLMs.” Not only is this a major leap in AI technology, but it’s also a game-changer for how AI models can dynamically adapt to tasks in real-time, and yes, the best part—it’s open source. Let’s unpack what makes this innovation so revolutionary.
◗ NOTE: This is an official Research Paper by “CLOXLABS“
◆ The Big Idea: Real-Time Model Updates
If you’ve followed the evolution of AI, you know one of the biggest challenges with traditional transformer architectures is their static nature. Once a model is trained, it’s essentially frozen in time. You can add knowledge through resource-intensive post-training methods like fine-tuning or Retrieval-Augmented Generation (RAG), but the model itself doesn’t learn or adapt during inference.
This is where “Transformer Squared” shines. SakanaAI introduces a self-adaptive framework that allows LLMs to update their weights at inference time based on the user’s query. This dynamic adaptability enables the model to better handle unseen tasks without requiring expensive re-training. Think of it as giving the model a surgeon’s scalpel, allowing it to make precise adjustments on-the-fly.
All Ads on this website are served by GOOGLE
◆ Breaking Down “Transformer Squared”
Let’s dive into how this system works, starting with its two-pass approach:
- First Pass: This stage analyzes the user’s prompt and identifies the task type—whether it’s math, coding, or logical reasoning. Essentially, it functions as a dispatch system to understand the nature of the query.
- Second Pass: Based on the first-pass analysis, the system selectively updates its weights using task-specific expert vectors. These vectors are pre-trained modules optimized for specific tasks, enabling the model to tailor its behavior dynamically.
The beauty of this method lies in its Singular Value Fine-tuning (SVF), a parameter-efficient technique that adjusts only the singular components of the model’s weight matrices. By targeting specific areas for modification, SVF avoids the inefficiencies of traditional fine-tuning, which often requires recalibrating large sections of the model.
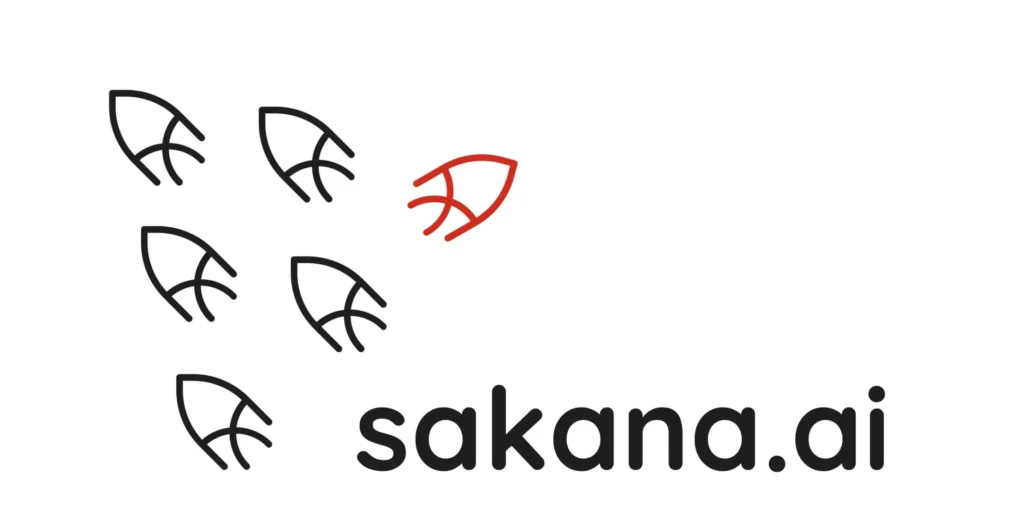
◆ Efficiency Meets Performance
One of the standout claims in the “Transformer Squared” paper is its efficiency. SakanaAI reports that their approach outperforms existing methods like LoRA (Low-Rank Adaptation) with far fewer parameters. The results suggest it’s not just about doing things better but also doing them smarter and faster.
For example:
- Task-specific Adaptability: Unlike static models, this system can handle a broader range of tasks without overfitting.
- Cost-Effectiveness: By focusing only on necessary updates, the model avoids the high computational costs associated with traditional fine-tuning.
- Reduced Memory Overhead: Dynamic updates eliminate the need for storing separate fine-tuned models for different tasks.
◗ Open Source and Cross-Domain Applications
SakanaAI has made the code for “Transformer Squared” publicly available, enabling its integration with other open-source models. While the primary focus has been on LLMs, the architecture has also demonstrated exceptional performance in vision models. This flexibility opens the door for cross-domain applications, from image recognition to complex multi-modal tasks.
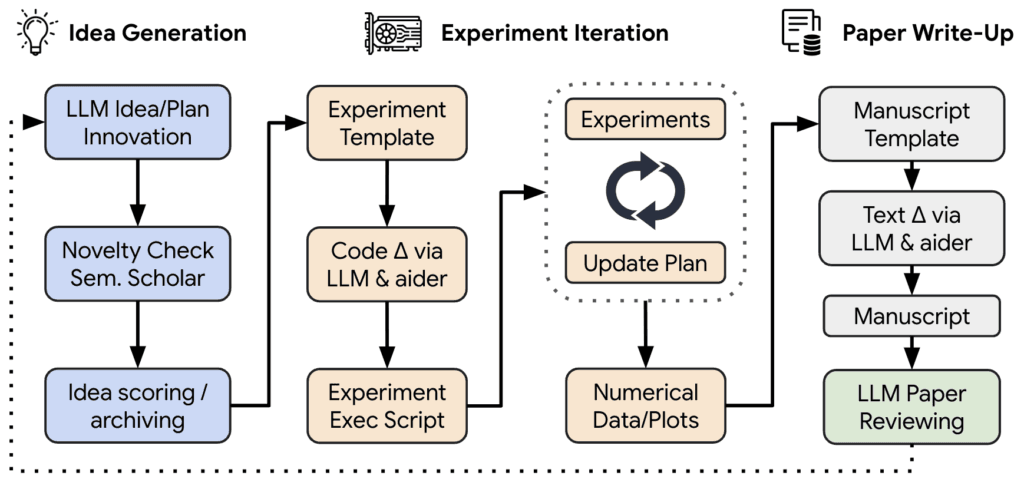
The underlying concept of “Transformer Squared” mirrors how the human brain works. Just as our brains activate specific regions based on the task at hand—writing, solving math, or creative thinking—this architecture uses expert modules that can be dynamically composed during inference. This modularity allows for continual learning without the risk of catastrophic forgetting, a problem common in traditional fine-tuning.
◗ Addressing Challenges in AI Training
The paper also highlights how “Transformer Squared” tackles some long-standing issues in AI training:
- Overfitting: By selectively fine-tuning only relevant parameters, the model minimizes the risk of overfitting, especially in narrow-task domains.
- Parameter Explosion: Expert modules are trained to be composable, reducing the overall number of parameters while maintaining high performance.
- Flexibility: The mixture-of-experts approach ensures the system can adapt to diverse tasks without constant re-engineering.
◗ Why This Matters: The Bigger Picture
The implications of “Transformer Squared” are profound. Not only does it redefine how we think about model adaptability, but it also signals a shift toward more sustainable AI practices. By optimizing computational efficiency, SakanaAI is addressing both economic and environmental concerns associated with large-scale AI deployments.
Moreover, this innovation aligns with broader trends in AI research, such as Google’s Titans Paper, which explores similar methods to make models more dynamic. As the industry moves toward less static, more real-time adaptable architectures, “Transformer Squared” positions SakanaAI as a leader in the next generation of AI development.
All Ads on this website are served by GOOGLE
Our Thoughts
“Transformer Squared” isn’t just an incremental improvement—it’s a paradigm shift. By enabling real-time updates during inference, SakanaAI has set a new benchmark for what AI can achieve. The open-source release ensures that this innovation isn’t limited to research labs but can be adopted and built upon by the global AI community.
Whether you’re an AI researcher, developer, or enthusiast, this is a moment to pay attention. SakanaAI isn’t just shaping the future of transformers; they’re shaping the future of AI itself.
All Ads on this website are served by GOOGLE
About the Author:
Amir Ghaffary – CEO of CLOXMEDIA – is on a relentless mission to revolutionize our grasp of the future, blending visionary insight with cutting-edge technology to craft a new paradigm of modern understanding. His work transcends traditional boundaries, bridging the gap between what is and what could be, inspiring a generation to rethink the possibilities of tomorrow. By advocating for a deeper integration of AI, digital transformation, and forward-thinking innovation, Amir is not just predicting the future—he’s actively shaping it, pushing society to embrace a bold new reality where technology and human potential are intertwined like never before.
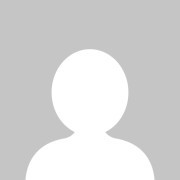
All Ads on this website are served by GOOGLE