Artificial intelligence (AI) has emerged as a game-changer in Personal Finance and Investing, reshaping how individuals budget, save, and grow their wealth. From intelligent budgeting apps to robo-advisors managing billions, AI is empowering both everyday consumers and finance professionals with data-driven insights and automation.
In the banking and financial services industry (BFSI) overall, the AI market was valued around $20 billion in 2022 and is projected to reach $100 billion by 2032, reflecting the rapid growth and investment in this technology. This research study by CLOXLABS explores how AI can be leveraged to enhance personal finance management and investment strategies, focusing on applications in the UK and US.
We will cover the current landscape of AI tools, real-world examples in both regions, and discuss the benefits and challenges of integrating AI into financial decision-making. enough of introduction… Let’s get into it but remember, the way we report it is more educational. We tried our best to make it understandable as much as we could.
NOTE: This is an official Research Paper by “CLOXLABS“
Overview:
AI’s Growing Role in Personal Finance & Investment
AI is increasingly embedded in the financial sector, powering solutions from virtual assistants to sophisticated trading algorithms. Modern AI techniques – including machine learning, natural language processing (NLP), and predictive analytics – enable financial firms and fintech startups to extract insights from vast data streams and make intelligent decisions with unprecedented speed and accuracy. Key areas of impact range from fraud detection to personalized financial advice. For example, AI-driven systems excel at spotting anomalies for fraud prevention, far outpacing traditional rule-based systems. On the customer side, AI is democratizing financial advice that was once “a privilege reserved for the wealthy” by delivering personalized guidance at scale, so that ordinary individuals can get tailored budgeting or investment advice once available only from human advisors. According to fintech research, the domains poised to benefit most from AI include automated trading and investment management, customer service chatbots, lending and credit scoring, as well as risk management and compliance.
In both the US and UK, banks and fintech companies are leveraging AI to enhance user experiences—examples range from AI assistants that handle everyday banking queries to robo-advisory platforms that optimize long-term investment portfolios. As Dr. Emily Johnson, Chief AI Strategist at FinTech Innovators, observes, “The convergence of AI and fintech is not merely a technological shift; it’s a paradigm shift that will redefine how we interact with and experience financial services. Those who embrace this revolution early and effectively will gain a significant competitive edge”. In the sections below, we delve into specific applications of AI in personal finance management and investing, illustrating the trends with UK and US examples.
All Ads on this website are served by GOOGLE
AI-Powered Personal Finance Management
Managing personal finances can be tedious and complex, but AI-powered tools are making it easier than ever to budget, track expenses, improve credit health, and automate savings. These tools act like always-on financial assistants, helping individuals make smarter money decisions daily. Below, we explore how AI enhances budgeting and expense tracking, credit score monitoring, and savings automation.
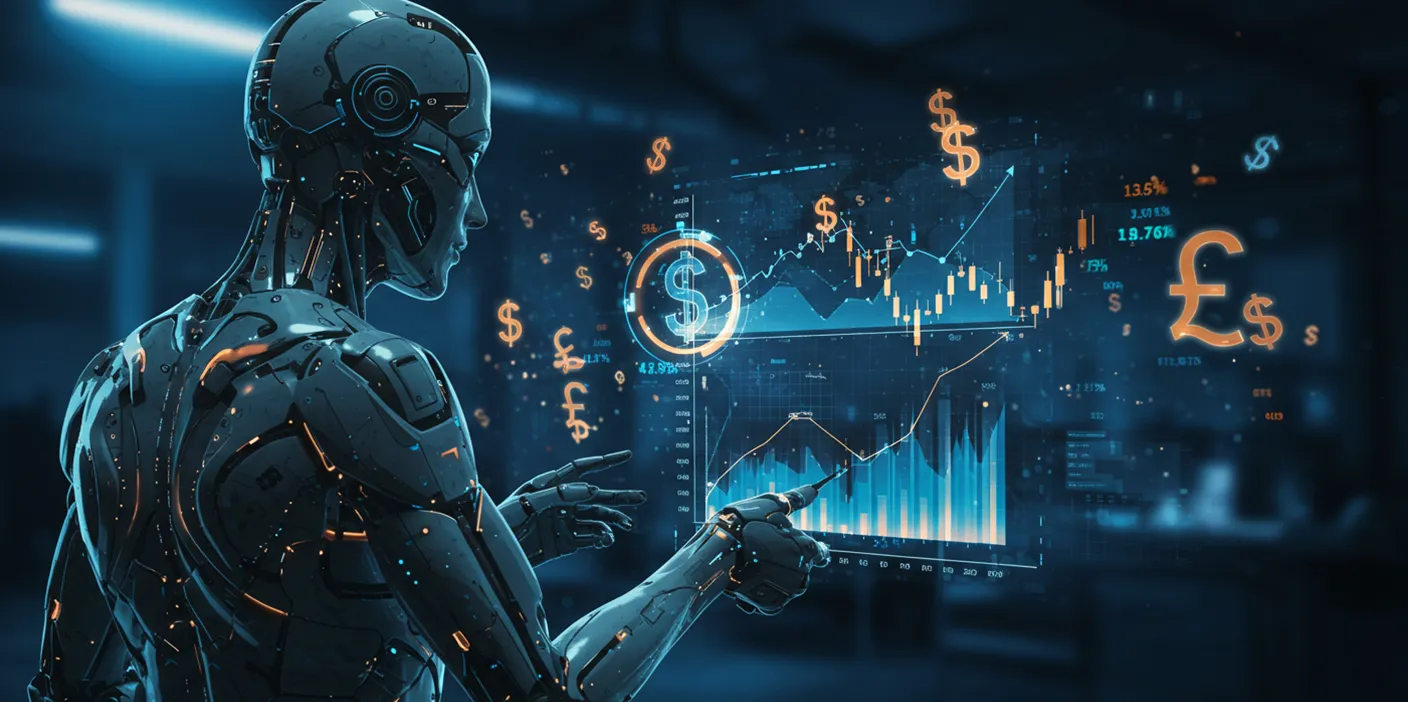
AI Budgeting and Expense Tracking Tools
One of the most accessible ways AI assists consumers is through smart budgeting apps and expense trackers. Instead of manually updating spreadsheets, users can rely on AI to analyze their bank transactions, categorize spending, and even receive personalized tips on saving. AI-driven budgeting apps like Wally and Cleo have gained popularity for their ability to provide insights with minimal effort from the user. For instance, Wally is an AI-powered app that automatically categorizes your income and expenses and then suggests when to save, even offering an in-app chatbot (“WallyGPT”) to simplify financial tracking. Similarly, the UK-born app Cleo presents itself as a chatty AI financial assistant that syncs with your bank accounts to create a personalized money management plan. Cleo’s AI analyzes your income, bills, and spending habits, then uses that data (plus a dose of humor) to nudge you toward better budgeting decisions – for example, activating a “roast mode” that playfully scolds you for overspending on takeaways.
These AI budgeting tools not only track past behavior but also learn from it: they can alert you of recurring subscriptions, project your month-end account balance, or identify categories where you consistently overspend. Bank of America’s virtual assistant Erica is another prominent example; it monitors customer finances and provides over 30 types of proactive insights, such as alerting users about upcoming bills or unusual spending. In fact, Erica has handled more than 2 billion client interactions to date, fielding about 2 million user queries per day on everything from budgeting help to transaction searches. This level of engagement shows how AI-driven personal finance tools have become like a “personal concierge” for millions of users, seamlessly embedded in their daily money management. Whether through standalone apps or bank-integrated assistants, AI budgeting tools in the US and UK are helping people gain control over their finances with less hassle and more personalization than traditional methods.
AI-Driven Credit Score Monitoring and Improvement
Maintaining a healthy credit score is a crucial part of personal finance, and AI is now being used to demystify credit scoring and coach users on improving their creditworthiness. AI-driven credit monitoring services can analyze a person’s financial behavior and forecast how certain actions (like paying down a balance or opening a new account) might impact their credit score in the future. Some banking apps even simulate “what-if” scenarios for users: for example, an app might show that increasing your monthly credit card payment by £50 could boost your score by a certain amount over six months.
On the lender side, AI-based underwriting models consider a wider range of data than traditional credit score formulas, which can benefit consumers with thin credit histories. In the US, online lender Upstart pioneered AI algorithms in lending decisions – using non-traditional data like education and employment – and reported that its AI model approved 44% more borrowers at 36% lower interest rates on average compared to a traditional credit model. This suggests AI can make credit more accessible and affordable by better distinguishing low-risk borrowers from high-risk ones. Similarly, credit bureaus are adopting AI to refine credit scoring; Equifax’s new OneScore platform employs machine learning to incorporate alternative data (e.g. utility payments, streaming subscriptions) into credit assessments, aiming for a more holistic and predictive measure of creditworthiness. For consumers, these advancements mean credit monitoring apps can give more tailored advice – for instance, recommending you pay down a specific debt first because the AI knows it will have the biggest impact on your score. Some apps will even alert you to harmful credit behaviors in real time. However, AI’s role in credit comes with a caution: it must be carefully managed to avoid encoding bias.
A notable case was the Apple Card incident in 2019, where a credit decision algorithm (developed by Goldman Sachs) was accused of offering significantly lower credit limits to women than men with similar profiles. The algorithm ostensibly “didn’t see gender,” yet the outcomes appeared discriminatory, prompting regulatory scrutiny. This underscores why transparency and fairness in AI credit models are so important – a theme we’ll revisit in the benefits and challenges section. Overall, though, when used responsibly, AI is proving effective in guiding individuals toward better credit and debt management, whether by providing actionable tips to raise a credit score or by enabling lenders to extend credit to more people on fair terms.
Smart Savings and Automated Financial Planning
Beyond budgeting and credit, AI is helping people save money and plan for the future with minimal effort through automated savings and financial planning tools. A great example in the UK is Plum, a smart money app that uses an AI algorithm to analyze your income and spending patterns and determine small, safe amounts to automatically transfer into savings or investments. Over 1.8 million users across the UK and EU have collectively set aside more than €2 billion using Plum’s AI-driven “autosave” feature. These algorithms act like a digital piggy bank that knows when you won’t miss a few extra pounds – it might save a bit more on a week you underspent on groceries, for instance, but hold off if it detects your balance is running low. Users remain in control by setting parameters (e.g. maximum to save per week), yet the day-to-day decisions are handled by AI. In the US, apps like Digit (now part of Oportun) and Rocket Money employ similar techniques, using machine learning to forecast upcoming expenses and identifying how much you can safely save without causing an overdraft. Rocket Money’s app analyzes your bills and subscriptions, then projects your cash flow to tell you how much “spendable” money you have left each month after obligations. This kind of insight is powered by AI parsing through your transaction data and learning your bill due dates, income schedule, etc., essentially doing forward-looking budget math for you.
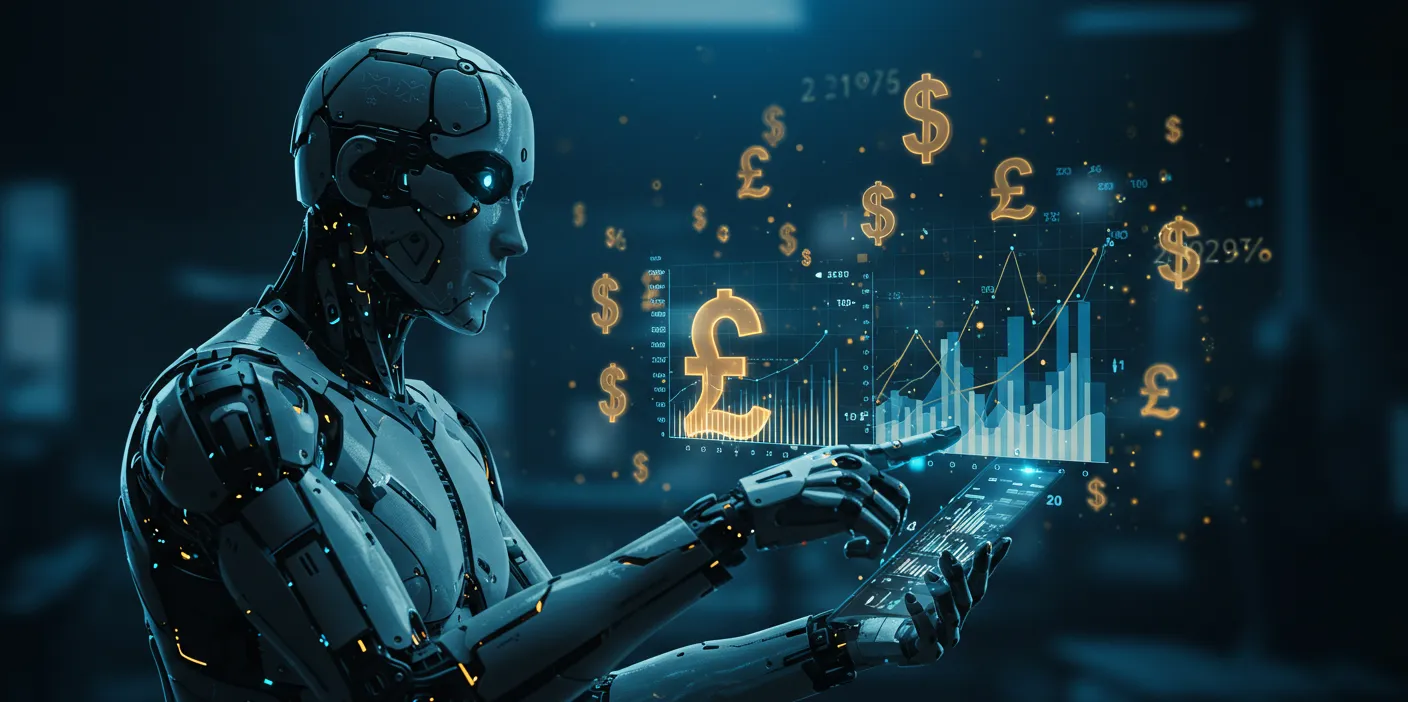
AI isn’t just for short-term savings – it’s also being used for long-term financial planning. Many robo-advisors and fintech apps now include goal-based planning modules that utilize AI to help users figure out how much to save or invest for a target (like buying a house or retirement). They can run simulations in the background; for example, an app might project that “if you continue saving £200 per month and investing with a moderate risk portfolio, there’s an 80% chance of reaching your £50,000 goal in 5 years.” These projections rely on Monte Carlo simulations or predictive models to account for market volatility and other variables. AI can quickly adjust the plan if the user’s circumstances change, offering actionable suggestions like increasing monthly contributions, when affordable or adjusting the investment mix. As an illustration, AI-driven platforms can “help customers understand potential outcomes based on simulations for different financial decisions, such as retirement planning or risk assessment,” which enables more informed decision-making. In both the UK and US, traditional institutions are also embracing AI for planning; robo-advisory services offered by banks often include planning tools that continuously adapt recommendations as the AI learns more about the client’s financial behavior. All of this means individuals can get a form of personalized financial planning without hiring a personal financial advisor – the AI quietly works in the background to optimize your saving and investing strategy over time.
All Ads on this website are served by GOOGLE
AI in Investment Strategies
Investing is another realm where AI is making a profound impact. Automated investing platforms (robo-advisors) and AI-assisted analytics are changing how portfolios are built and managed, while algorithmic trading bots (especially in the high-paced cryptocurrency markets) execute strategies with superhuman speed and data awareness. This section examines how AI is powering robo-advisors, enhancing stock market analysis, and driving new approaches to trading, with an eye on trends in the US and UK.
Robo-Advisors and Automated Investment Platforms
Robo-advisors have become emblematic of AI in consumer investing. These are online platforms that use algorithms to invest your money based on your goals and risk tolerance, typically at a fraction of the cost of traditional human financial advisors. By asking users a few questions about their financial goals and risk comfort, robo-advisors can allocate investments across diversified portfolios (usually composed of ETFs and index funds) and automatically rebalance those portfolios over time. The appeal is the combination of convenience, low fees, and evidence-based strategies. Unlike human advisors, robo-advisors are available 24/7 and often require little to no minimum investment, making wealth management more accessible; plus, they charge much lower fees (sometimes 0.25% of assets per year, versus 1% or more for a human advisor). The US has led in robo-advisor adoption – for example, Betterment, one of the early robo-advisory firms, now manages over $50 billion in assets for more than 900,000 clients as of 2024. Vanguard, Schwab, and other incumbents have also rolled out robo or hybrid advisory services that collectively serve millions. The growth isn’t limited to the US: UK-based robo-advisors are on the rise as well. Nutmeg, a UK digital wealth manager founded in 2012, amassed about 140,000 clients and £3.5 billion in assets under management by 2021, becoming one of Britain’s best-known robo-advisors. Its success prompted an acquisition by JP Morgan Chase to integrate Nutmeg into the bank’s international digital offering. This underscores how major financial institutions view AI-driven investment platforms as critical to the future of wealth management. Globally, the assets under management by robo-advisors are projected to reach around $2 trillion in the mid-2020s, up from just $22 billion in 2019. Figure: Assets under management (AUM) of robo-advisors worldwide have skyrocketed in recent years. The chart above illustrates the growth trajectory, rising from an estimated $22 billion AUM in 2019 to over $1.3 trillion in 2023, with forecasts of roughly $2.3 trillion by 2028. This explosive growth in AUM reflects both the popularity of robo-advisors among investors and the trust being placed in algorithm-driven strategies.
Robo-advisors use AI in various parts of their operation. Portfolio recommendations may be optimized through machine learning based on outcomes of countless portfolio scenarios. Some platforms like Magnifi even incorporate conversational AI, letting users ask questions via a chat interface to explore investment options. As these platforms evolve, we also see a trend toward hybrid models – combining automated portfolios with access to human advisors for more complex questions – effectively using AI to do the heavy lifting while humans provide a personal touch.
This hybrid approach is promoted as the best of both worlds: the AI handles data-crunching and routine rebalancing, and a human advisor steps in for nuanced guidance, tailoring the strategy to the client’s unique circumstances and emotions. Whether fully automated or hybrid, AI-powered investment platforms are becoming mainstream in both the US and UK, giving a wider population the benefit of sophisticated portfolio management and financial advice once reserved for high-net-worth individuals.
All Ads on this website are served by GOOGLE
AI-Assisted Stock Market Analysis and Predictive Analytics
Investors have always sought an edge in the stock market, and AI is providing new tools to analyze market trends, company fundamentals, and even social media sentiment to inform trading decisions. AI-assisted stock analysis platforms use machine learning models to process vast amounts of financial data far beyond human capacity. They can digest news articles, earnings reports, historical price trends, and macroeconomic indicators to detect patterns or make predictions about future movements.
For example, some AI models are trained to identify early warning signals in stock price movements or to forecast volatility, which can be incredibly valuable for both individual traders and institutional investors. In fact, the majority of trading activity in major financial markets is already driven by algorithms – studies estimate that 60–80% of trading volume in equities and forex markets comes from automated trading bots rather than manual trades. These algorithms range from simple rule-based systems to complex AI agents that learn and adapt. For retail investors, fintech startups have started offering AI-powered analytical tools: one platform might use neural networks to rank stocks based on projected return vs. risk, while another might employ NLP to gauge market sentiment from Twitter or Reddit posts (trying to catch the next “meme stock” wave or avoid it). There are even exchange-traded funds (ETFs) managed by AI – for instance, an AI-driven ETF in the US uses IBM Watson technology to pick a portfolio of stocks dynamically, updating its holdings based on AI’s interpretation of myriad data points.
While fully autonomous AI stock picking for retail users is still emerging, AI-driven insights are increasingly embedded in consumer trading apps. Many brokerage platforms now offer AI-generated alerts like “this stock’s sentiment is very positive today” or “your portfolio is exposed to X risk factor based on AI analysis,” which help users make more informed choices.
It’s worth noting that predictive analytics in investing is as much about managing risk as chasing returns. AI can run simulations and stress tests on portfolios extremely quickly – for example, showing how a portfolio might perform if interest rates rise by 1%, or if a 2008-like crisis occurred again – thereby helping investors (or their robo-advisors) adjust strategies proactively. Large banks and hedge funds in the US and UK invest heavily in AI to support their trading strategies, using techniques like reinforcement learning to optimize trading algorithms over time. These advanced systems might remain proprietary, but their techniques trickle down to everyday tools. In practice, an individual investor might use an AI-driven screener to identify undervalued stocks, or use an app where they can ask a ChatGPT-like advisor, “What’s the outlook for tech stocks this quarter?” and get a synthesized analysis drawn from recent market data (a scenario that may not be far off, given the rapid development of GPT-style financial assistants).
While no AI can predict markets with certainty – and investors should remain cautious because markets can behave in ways models don’t expect – AI’s ability to parse complex data can give investors a better informational foundation for their decisions. Indeed, some studies claim AI-based trading strategies can outperform manual ones by 10–30% when configured well, particularly in fast-moving markets. As the technology matures, we can expect AI-driven analysis to become a standard feature of investment research, both at the professional level and in consumer finance apps.
Cryptocurrency Trading Bots and AI Agents
The cryptocurrency market is a space where AI-driven trading has particularly taken hold. Crypto trades 24/7, is notoriously volatile, and is largely digital-native, making it fertile ground for algorithmic and AI trading bots. In the early days of crypto, traders used simple bots to do things like arbitrage (buy on one exchange, sell on another) or to execute predefined strategies even while they slept. Now, more advanced AI agents are being deployed to navigate the wild swings of Bitcoin, Ethereum, and countless altcoins. These AI agents leverage machine learning to adapt their strategies in real-time, reacting to new data such as sudden news events, changes in trading volume, or shifts in investor sentiment across social media. Unlike basic bots that follow fixed if-then rules, AI-driven bots can modify their own trading criteria on the fly – for example, if an AI agent “learns” that a certain pattern in price and volume often precedes a rally, it can start to exploit that pattern until it no longer works. This adaptability is crucial in crypto markets; as one analysis put it, traditional bots can struggle when conditions change, but AI agents with self-learning capabilities can adjust to “offer adaptability, contextual understanding, and self-learning” when making trading decisions.
Both the US and UK have active communities of crypto traders using AI tools. There are cloud-based services where users can rent AI trading algorithms or even train their own on historical data. Some high-frequency trading firms in crypto have AI engines processing millions of data points per second to capture short-lived opportunities (for instance, detecting a big buyer in the market and riding the momentum for a few seconds). Reports often cite that in crypto, well-tuned bots can yield higher returns than manual trading, though success is far from guaranteed and the risks are significant. Importantly, AI is also being used for risk management in crypto – such as algorithms that can flag when a user’s portfolio is over-leveraged or at risk of liquidation in real-time, allowing proactive adjustments.
One fascinating development at the intersection of AI and crypto is the use of sentiment analysis and social data to trade assets. Crypto prices can be heavily influenced by online discourse (a single Elon Musk tweet can send Bitcoin soaring or plummeting). AI systems ingest Twitter feeds, Reddit threads, and news headlines, attempting to quantify the market mood and anticipate moves. For example, an AI might detect an uptick in positive mentions of a smaller cryptocurrency and decide to buy in before the price potentially jumps. Conversely, it might catch wind of regulatory rumors or negative news early and exit positions to avoid losses. Such AI-driven responsiveness is something human traders struggle to match at scale.
All Ads on this website are served by GOOGLE
However, crypto AI bots also illustrate the caveats of AI in finance: if not properly configured, they can make bad decisions quickly and at scale. There have been instances of bot algorithms malfunctioning or overreacting to false signals, leading to flash crashes or bad trades. Therefore, many crypto traders run bots on a short leash – monitoring them or using circuit-breakers to shut them off if things go awry.
In summary, AI is increasingly integral to crypto trading for those seeking an edge, handling everything from routine arbitrage to complex strategy optimization. As more retail investors dip toes into crypto, we’re seeing more user-friendly AI trading tools tailored to non-experts as well, sometimes packaged as “robo-advisors for crypto.” Whether in traditional stocks or digital assets, AI’s ability to operate at high speed and analyze diverse data is changing how trading is done, but it comes with a learning curve and the need for oversight.
Case Studies & Real-World Applications in the UK and US
To illustrate AI’s impact on finance in practice, let’s look at several real-world applications and success stories from both the UK and US. These cases highlight how both fintech startups and established financial institutions are leveraging AI to deliver better financial tools and outcomes for users.
◗ Bank of America’s Erica (US) – One of the most successful AI-driven personal finance tools is Erica, the virtual financial assistant in Bank of America’s mobile app. Launched in 2018, Erica uses AI and NLP to help customers with everyday financial tasks. It can do things like provide balance updates, send spending insights, assist with bill reminders, and answer questions like “How much did I spend on groceries last month?”.
Erica has been rapidly embraced by customers: as of 2024, over 42 million clients have used it, and it has handled more than 800 million inquiries in total. Clients now engage with Erica about 2 million times each day, asking for assistance or receiving proactive tips. The assistant grew from 1 billion interactions to 2 billion in just 18 months, showing accelerating adoption. Importantly, Erica doesn’t just wait for questions – it provides over 30 types of proactive insights. For example, it monitors recurring charges and will notify a user if a free trial is about to turn into a paid subscription (Erica sends 2.6 million such subscription alerts per month). It also analyzes spending patterns to give feedback (2.2 million insights on spending behavior per month) and keeps users informed of unusual account changes like duplicate charges or unexpected fees. These features effectively turn the bank app into a financial coach.
Bank of America attributes Erica’s value to “applied innovation in language processing and predictive analytics to deliver a valuable and empowering client experience,” and it has become a template that other banks around the world are studying. The success of Erica demonstrates how a well-designed AI assistant can deepen customer engagement and satisfaction in personal finance by making banking more interactive and insight-driven.
◗ Cleo – AI Budgeting Chatbot (UK/US) – Cleo is a London-founded fintech startup that embodies AI’s potential in personal finance, particularly for younger generations. Cleo began as a chatbot on Facebook Messenger in 2016, and has since evolved into a standalone app with a distinct personality. It targets Millennials and Gen Z users with a conversational interface that makes managing money feel more approachable (and even fun). Cleo’s AI connects to users’ bank accounts via open banking APIs and analyzes their transactions to give personalized budgeting advice and savings tips. The twist is Cleo’s tone – it’s witty, sometimes sassy. If you give Cleo permission, it will playfully call you out for bad spending habits (its infamous “roast mode”), or cheer you on when you hit savings goals.
This gamified, engaging approach has proven popular: Cleo has amassed over 7 million users globally and reached $150 million in annual recurring revenue as of late 2024. While Cleo started in the UK, it focused on the U.S. market early for growth and is now planning an expanded UK presence again. Beyond budgeting help, Cleo also offers features like salary advances and a credit builder product, indicating how AI-based insights can be extended into financial services traditionally offered by banks. The company employs a combination of large language models (LLMs) and rule-based AI to drive the chatbot, and even has comedians on staff to keep Cleo’s persona fresh with relevant jokes and cultural references. Cleo’s success story shows a fintech effectively blending AI technology with user-centric design – addressing serious matters like saving money, but in a relatable way that resonates with millions. It also highlights the transatlantic nature of many fintech innovations (born in the UK, scaling in the US, in this case) and how AI can be used as a differentiator to attract users in a crowded personal finance app market.
◗ Nutmeg and Betterment – Robo-Advisor Growth (UK & US) – As discussed earlier, robo-advisors have become prominent in real-world investing. Betterment in the US and Nutmeg in the UK serve as two case studies on each side of the pond. Betterment’s journey (with $50B+ AUM and nearly a million customers) shows how American investors have warmed to automated investing—many using it for goals like retirement or general wealth-building. Betterment’s platform continuously uses AI techniques to optimize tax efficiency (e.g., tax-loss harvesting algorithms) and personalize advice (recently integrating human advice options).
Meanwhile, Nutmeg’s rise in the UK – growing into one of the largest UK digital wealth managers with £3.5 billion AUM… illustrates both the opportunity and challenges for robo-advisors in Britain. Nutmeg proved that UK investors would trust an online, AI-driven service with their money, paving the way for incumbents and new players to launch similar services. Its acquisition by JPMorgan Chase in 2021, to form the basis of the bank’s international digital investing offering, validates the robo-advisor model and shows how traditional banks are embracing fintech innovations via partnerships or takeovers. Post-acquisition, Nutmeg has continued to operate under the Chase brand, and large UK banks like Barclays and HSBC have also begun offering or exploring automated investment products. The key takeaway from these cases is that AI-driven investment platforms have achieved real scale. They have moved from niche startups to mainstream adoption, and their influence is prompting the broader wealth management industry to adapt (for instance, many human financial advisors now use AI-based software to enhance their own advice). For consumers in the UK and US, this means more choices and lower costs when it comes to investing, as competition heats up between robo-advisors and traditional services.
◗ AI in Credit and Lending – Fairness and Inclusion – Another real-world application of AI is in lending, where it’s used to expand credit access responsibly. We touched on Upstart’s model earlier as a success story: by leveraging AI for underwriting, Upstart helped banking partners issue 27% more loans than they would have under traditional criteria, with lower default rates, according to data shared with the U.S. Consumer Financial Protection Bureau【19†L33-L40** (Upstart’s model approved significantly more minority borrowers as well, while reducing interest rates)【20†L205-L214】. In the UK, large lenders are also trialing AI to make credit decisions more inclusive – for example, using AI to incorporate rental payment history into mortgage lending decisions, which could help younger borrowers who have good rental track records but no prior mortgage. Credit bureaus like Experian have introduced services like Boost (which lets consumers add utility and phone payments to their credit file) aided by algorithms that determine how much those behaviors improve one’s score. And as noted, Equifax’s OneScore ML model is a concrete step toward a more AI-driven credit scoring system.
However, real-world use has also surfaced challenges: the Apple Card algorithm issue in 2019 became a high-profile example of how an opaque AI model can lead to public outcry and regulatory questions if outcomes seem unfair. This led to an investigation in New York and spurred broader discussions on “algorithmic accountability” in financial services. In response, many institutions are now more transparent about their AI use, often touting independent audits or bias testing of their models. The UK’s Financial Conduct Authority (FCA) and the US CFPB have both indicated they are watching AI in finance closely to ensure it doesn’t harm consumers. These case studies collectively show that AI is not just theoretical in finance; it’s out in the real world handling people’s money. The successes are impressive – from massive user engagement with bank chatbots to startups achieving unicorn status by solving financial problems with AI. Yet each success is tempered by lessons learned on the importance of oversight, fairness, and earning user trust. Next, we’ll weigh the general benefits and challenges that come with deploying AI in personal finance.
Engineered ChatGPT Prompts for Financial Strategies
One exciting development in AI for personal finance is the use of advanced language models like ChatGPT to act as interactive financial assistants. By crafting effective prompts, users can leverage ChatGPT (or similar AI) to help with budgeting, investing research, and market analysis in a conversational manner. Below are examples of engineered prompts by CLOXLABS for you – carefully structured queries – that illustrate how one might use an AI chatbot to optimize financial planning and strategy. These prompts are designed to be technical and detailed to elicit sophisticated, useful responses from the AI:
◗ Budgeting Assistant Prompt: “You are an AI financial planner. I will provide you my monthly income, expenses, and savings goals. Analyze my spending categories and identify areas where I can cut costs. Then, create a optimized monthly budget for me following the 50/30/20 rule (50% needs, 30% wants, 20% savings), adjusted to reach my goal of saving an extra £200 per month. Provide the new budget breakdown and two specific tips for reducing expenses in my highest-spend categories.”
What it does: This prompt sets up ChatGPT to take on the role of a personal budgeting coach. It asks for analysis (finding where the user overspends) and application of a known budgeting framework (50/30/20 rule) tailored to the user’s goal. A good AI response would perhaps list the current vs. suggested spending in categories (housing, food, entertainment, etc.) and give tips like “swap Gym X for a cheaper Y” or “set a cap on dining out.”
◗ Investment Portfolio Analysis Prompt: “Act as a financial analyst with expertise in portfolio management. I have a portfolio of 60% stocks (broad index funds), 30% bonds, and 10% cash, but I’m concerned about risk and recent market volatility. Given the current economic climate (rising interest rates and inflation around 3%), provide an analysis of my portfolio’s risk factors. Suggest a reallocation strategy using principles of Modern Portfolio Theory to achieve a more conservative 50% stocks, 40% bonds, 10% cash allocation. Explain the expected impact on my portfolio’s risk/return profile and why this allocation is suitable now.”
What it does: This prompt asks ChatGPT for a relatively advanced task: analyze a portfolio in context of economic conditions and apply finance theory (Modern Portfolio Theory). The expected answer would discuss how rising interest rates might affect bonds and stocks, the notion of diversification, and the trade-offs in shifting to more bonds (lower expected return but lower volatility). It would then recommend specific changes (like moving 10% of stocks into bonds) and justify them in plain language.
◗ Stock Market Analysis Prompt: “You are an AI investment research assistant. I will give you three stock tickers and some recent news headlines for each. Provide a comparative analysis of these stocks. For each company, summarize what the news suggests about its outlook (e.g., growth prospects, challenges) and any notable recent financial performance. Then, based on this analysis and general market trends, rank the stocks from most promising to least for a 1-year investment horizon. Justify your ranking with data points or reasoning from the news.”
What it does: Here the prompt sets up a task combining news analysis and stock evaluation. The user would feed in, for example, “AAPL: (headline 1), (headline 2)…; GOOGL: (headlines)…; TSLA: (headlines)…”. The AI is asked to parse qualitative information (headlines) and quantitative if available (financial performance hints) and form an opinion. A well-constructed response would note things like “Apple’s news shows strong iPhone sales (growth prospects good), Google is facing an antitrust lawsuit (challenge), Tesla had record deliveries but also recalls (mixed outlook).” Then it might rank say Apple > Tesla > Google for the next year, with reasons. This kind of prompt shows how even a novice investor could use AI to digest financial news and get a synthesized analysis that would normally require an equity research background.
◗ Cryptocurrency Trading Prompt: “Pretend you are a crypto trading bot with AI capabilities. I’m interested in Bitcoin (BTC) and Ethereum (ETH). Analyze the current market situation: recent price momentum, trading volume spikes, and any notable news (like regulatory developments or major adoptions). Based on this, suggest a short-term trading strategy for the next month for BTC and ETH. Include entry and exit price targets and a brief risk assessment (e.g., how you’d adjust if the market turns bearish).”
What it does: This prompt is tailored for crypto enthusiasts. It asks the AI to consider multiple factors (price momentum, volume, news) and come up with a concrete trading plan. While ChatGPT can’t truly predict prices, an ideal answer would outline something like “Bitcoin has bullish momentum after breaking $X level with high volume, suggest buying on any dip near $Y and taking profit at $Z, but set a stop-loss at $W in case of bearish reversal due to regulatory news… Ethereum similarly…” along with caveats about volatility. Essentially, it forces the AI to articulate a plausible strategy and risk management approach, which a user could then evaluate.
:: OMG ::
Introducing “Million Dollar Worth of ChatGPT Prompts”
Now you understand the significance of structured prompts. Let us discuss how you can access them. This is a prompt set we developed with suggestions from various content creators, which is further accompanied by testing. This set comprises +60 prompts, and we call it “Million Dollar Worth of ChatGPT Prompts”. The aim of the package is to help you achieve the following:
⬤ Make money out of your work ⬤
⬤ Enhance your brand and articulate voice ⬤
⬤ Monitor your metrics for growth ⬤
⬤ Cut down the time spent without compromising on quality ⬤
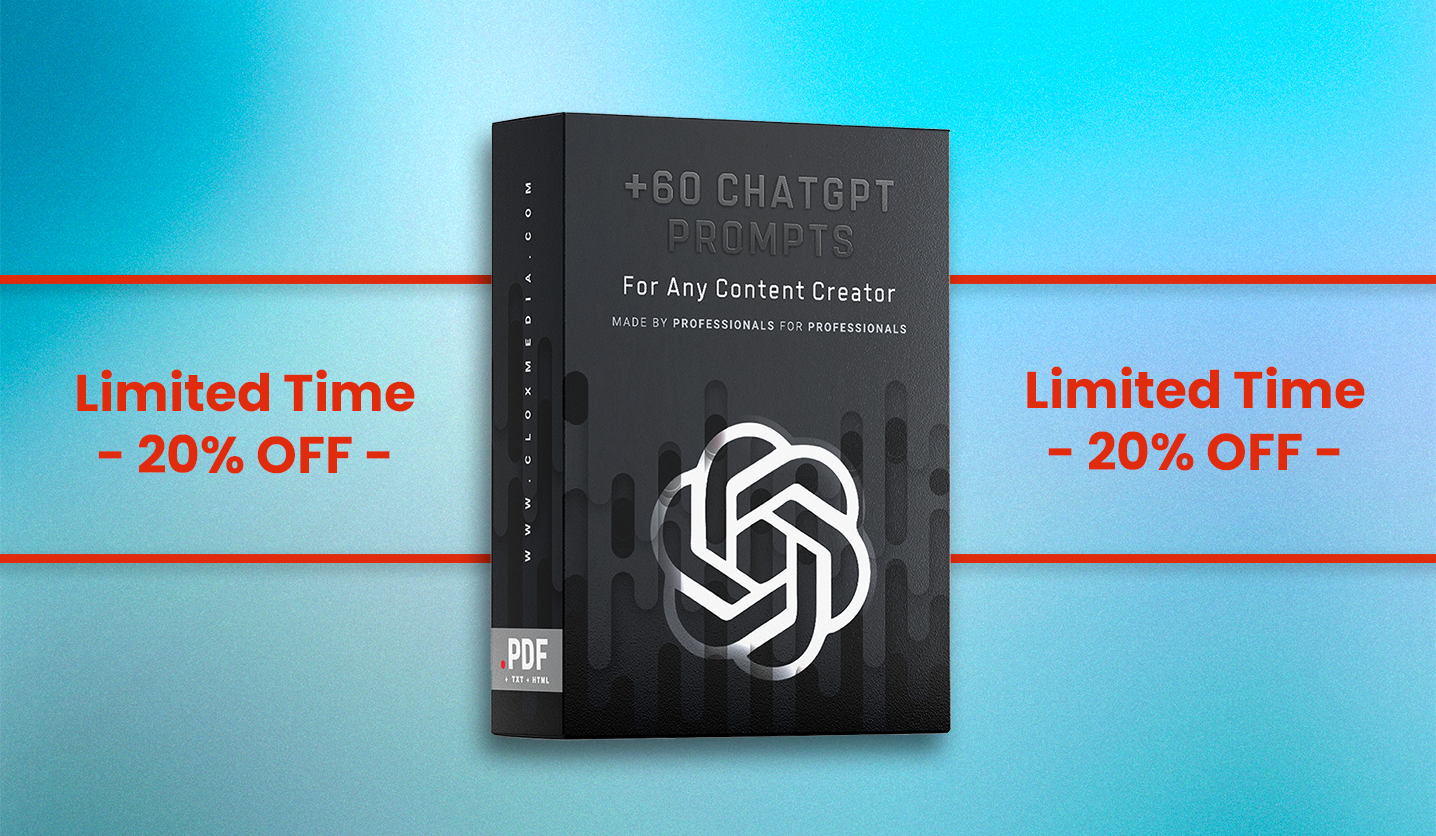
These prompts are not some vague random tips; instead, they are highly insightful pieces of information that focus on boosting engagement and reaching more people through your content to make more money.
When using such prompts, it’s important to remember that ChatGPT does not have real-time data or the ability to truly predict future market movements. However, these engineered prompts show how one can get analysis, scenario planning, and educational explanations from an AI assistant that can inform your strategies. Financial professionals might use prompts like these to generate first-draft analyses or to double-check their reasoning, while beginners can use them to learn and explore what questions to ask and what factors to consider. Always, any AI-generated suggestions should be considered critically and supplemented with professional advice when needed, but the ability to converse with an AI about finance opens up a powerful avenue for learning and decision support.
CLOXMAGAZINE, founded by CLOXMEDIA in the UK in 2022, is dedicated to empowering tech developers through comprehensive coverage of technology and AI. It delivers authoritative news, industry analysis, and practical insights on emerging tools, trends, and breakthroughs, keeping its readers at the forefront of innovation.
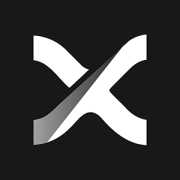