With the recent introduction of their reasoning models o3 and o4-mini, OpenAI has once again advanced the field of artificial intelligence. These new additions to the AI reasoning models have attracted a lot of attention from both professionals and industry insiders. However, what is so special about them and which one is more worthy in terms of attention or budget? I personally undertook the task of testing both models for several hours and I am now ready for this review, devoid of marketing fluff and emphasizing on what these AI models are useful for.
NOTE: This is an official Research Paper by “CLOXLABS“
The New Kids on the AI Block
Do you remember when ChatGPT was first released and it was absolutely mindblowing? Well, OpenAI’s recent models outdo that in every single way. The o3 model is said to be OpenAI’s premium reasoning model which is a real computing beast for multi-step, deep-thought processes and problem-solving. Its younger sister, o4-mini, came out only recently as a faster and cheaper model that does not sacrifice much power.
These models are part of the “o-series” by OpenAI, which, unlike the GPT catalog, is centered on reasoning capabilities. They are trained to pause prior to responding and utilize multiple methods to enhance their problem-solving skills. Their multimodal capabilities are astonishing – they can understand and interpret text, code, and images with effortless coordination.
The reasoning capabilities in the o3 model have increased markedly, making it suitable for high-end users with great financial resources, thus justifying the label reasoning flagship from OpenAI. The same cannot be said for o4-mini, which bears the distinct purpose of providing access to advanced reasoning for a greater number of users and applications at almost every level.
Under the Hood: Capabilities and Specifications
Let’s talk nerdy for a minute. Both models come with impressive specs that would make any tech enthusiast drool. They share a generous 200,000-token context window and can generate responses up to 100,000 tokens long. This means they can digest entire research papers, codebases, or complex instructions and produce comprehensive outputs in a single go.
Tool integration is where these models truly shine. Both o3 and o4-mini support Python execution, web browsing, and image analysis right out of the box. This allows them to:
- Run code to solve mathematical problems or analyze data
- Search the web for up-to-date information
- Process and understand visual information
- Generate comprehensive reports combining multiple data sources
The difference comes in how they process these tasks. O3 takes more time to think through problems, often producing more precise answers for complex questions. It excels in scenarios requiring intricate reasoning chains or nuanced understanding. I noticed this particularly when working with abstract mathematical concepts or complex coding challenges.
O4-mini, on the other hand, aims for efficiency without major compromises. It processes requests faster and with less computational overhead. Interestingly, it comes in two flavors: regular o4-mini and o4-mini-high. The high version spends more time on internal processing, producing higher-quality outputs for complex tasks, but at the expense of speed.
Battle of the Benchmarks: How Do They Stack Up?
Numbers don’t lie, and the benchmark results for these models tell an interesting story. In math reasoning tests like AIME 2024 and 2025, o4-mini actually outperformed o3, scoring 93.4% and 92.7% respectively, compared to o3’s 91.6% and 88.9%. This surprising result challenges the assumption that bigger always means better.
For coding tasks, the playing field becomes more level. On the Codeforces benchmark, o4-mini scored slightly higher than o3 with an ELO of 2719 compared to o3’s 2706. However, in more practical software engineering tests like SWE-Bench Verified, o3 maintained a slim lead with 69.1% versus o4-mini’s 68.1%.
Where o3 pulls ahead more decisively is in the Aider Polyglot code editing benchmark, scoring 81.3% (whole) and 79.6% (diff) compared to o4-mini-high’s 68.9% and 58.2%. This suggests that for complex code editing and generation, o3’s additional reasoning capabilities provide tangible benefits.
I personally tested both models on a simple yet confusing math problem, and the results were illuminating. O4-mini initially provided an incorrect answer but corrected itself when I suggested using a calculator tool. O3, while slower to respond, produced the correct solution on the first attempt with clearer reasoning steps.
For visual tasks, I was surprised to learn that according to an OpenAI insider, o4-mini is actually a better vision model than o3. This was evident when I tested both models on image analysis tasks – o4-mini provided more accurate descriptions and insights from visual inputs, despite being the “mini” version.
The Price of Intelligence: Cost Considerations
Here’s where things get really interesting – and where most users will make their decision. The price difference between these models is staggering. O3 charges $10.00 per million input tokens and a hefty $40.00 per million output tokens. By comparison, o4-mini is practically a bargain at just $1.10 per million input tokens and $4.40 per million output tokens.
To put this in perspective, that’s roughly a 10x price difference across the board. For large-scale applications or extensive personal use, this can translate to thousands of dollars in savings while still getting comparable performance on many tasks.
Beyond the raw costs, there are usage limits to consider. Consumer accounts can send up to 50 messages per week to o3, while o4-mini allows for a more generous 150 messages per day. This makes o4-mini not just cheaper, but more accessible for frequent users.
During my testing, I found that o4-mini was not just adequate but exceptional for most everyday tasks. I used it to analyze research papers, generate code, explain complex concepts, and even create visual content. For most of these applications, I couldn’t justify the additional expense of o3, especially when running multiple queries.
Real-World Application: When to Choose Which Model
After spending considerable time with both models, I’ve developed a simple framework for deciding which one to use:
Choose o3 when:
- You’re working on mission-critical tasks where precision is paramount
- You need to generate or debug complex code with many interdependencies
- You’re dealing with highly scientific or academic content requiring nuanced understanding
- Budget is less of a concern than getting the absolute best results
Choose o4-mini when:
- You need fast responses for real-time applications
- You’re processing large volumes of content
- You’re working with visual inputs (where it surprisingly outperforms o3)
- You want to optimize for cost efficiency without major performance sacrifices
- You need more daily usage capacity
For many professional applications, I found that o4-mini-high provides the sweet spot – better quality than regular o4-mini but at a fraction of o3’s cost. This configuration works particularly well for content creation, research assistance, and educational applications.
Looking Beyond the Specs: The Human Experience
Numbers and benchmarks only tell part of the story. What really matters is how these models perform in real-world scenarios. During my extensive testing, I found both models to be significantly more helpful than previous generations of AI assistants.
The reasoning capabilities make conversations feel more natural and productive. Rather than providing superficial answers, both models work through problems methodically, often catching their own mistakes and correcting course. This makes them feel more like collaborators than tools.
For creative tasks like generating a simple game, I found that o4-mini-high produced results comparable to other leading models like Gemini 2.5 Pro. The code was clean, well-structured, and demonstrated understanding of both technical requirements and creative direction.
When analyzing complex data or research papers, both models excelled at extracting insights and presenting them in accessible formats. O3 provided deeper analysis with more nuanced understanding of implications, while o4-mini delivered cleaner, more straightforward summaries that were easier to act upon.
Conclusion: Revolution or Evolution?
After weeks of testing these models across countless scenarios, we’re convinced that OpenAI’s o-series represents a significant step forward for AI reasoning capabilities. These aren’t just incremental improvements – they fundamentally change what’s possible with artificial intelligence.
O3 stands as the premium option for those who need the absolute best in reasoning capabilities and are willing to pay for it. It’s the Formula 1 car of AI models – expensive, sometimes excessive, but unmatched when you need peak performance.
Meanwhile, o4-mini delivers perhaps the more impressive achievement – bringing near-flagship level reasoning to a much wider audience through dramatically lower pricing. It’s like getting a sports car for the price of a sedan, with only minor compromises in performance.
For most users and applications, o4-mini represents the better value proposition. Its combination of speed, affordability, and capability makes it suitable for everything from personal use to enterprise applications. The optional high-inference configuration provides flexibility when you need additional reasoning power.
But the real winner here is us – the users. These models represent a new era of AI assistants that can truly reason through problems rather than simply pattern-matching responses. Whether you opt for the premium o3 experience or the more accessible o4-mini, you’re getting a glimpse of what the future of AI looks like – and it’s both impressive and a little intimidating.
As these models continue to evolve, the line between human and machine reasoning grows increasingly blurred. The question is no longer if AI can reason, but rather how we’ll harness these new capabilities to solve problems that matter. And that, more than any benchmark or specification, is what makes this technology truly revolutionary.
CLOXMAGAZINE, founded by CLOXMEDIA in the UK in 2022, is dedicated to empowering tech developers through comprehensive coverage of technology and AI. It delivers authoritative news, industry analysis, and practical insights on emerging tools, trends, and breakthroughs, keeping its readers at the forefront of innovation.
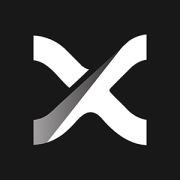