As an AI expert from CLOXLABS, I have seen many AI models come and go, each with its own swag of improvements (and sometimes letdowns). Taboos are Shattering, there is a substantial shift away from the survivi g Transformer type of model when it comes to didactic frameworks in the case of Liquid AI’s Liquid Foundation Models (LFM). Our emphasis is mainly focused on those who are stuck in the quagmire of this term generative AI. This has been a bold one. And it poses a number of interesting questions on the development of generative AI in the future.
NOTE: This is an official Research Paper by “CLOXLABS“
— Electrical Shock:
Why is this even a break from Transformers?
LFM model came in three different sizes- 1 billion, 3 billion, and 40 billion parameters. most notable enticing factor of these models other than their impressive performance is the efficiency within their memory. Liquid AI asserts that these tokens outputs requiring such models can be done with considerably lesser memory than how other models of the same class opt for. In this day and age, where computing resources and memory capacity is beginning to be a considered issue, the seeing is believed to be…
Indeed, several years later, the Transformer became the go- to design principle of modern AI models: a design responsible for the success of models such as GPT, BERT, LLaMA etc. Innova- tions are therefore expected with every new model that emerges. It should however be noted that whenever a new architecture joins the fray especially one that pawns the efficient and proficient transformer one, it captures the brain. Liquid AI`s new LFM models do just that.
All Ads on this website are served by GOOGLE
— Benchmarks:
Numbers Tell, But Do They Tell The Whole?
Let’s discuss performance. Based on the benchmarks done by Liquid AI, the LFM models are better than quite a few well-entrenched competitors like LLaMA and Jamba, especially the MMLU Pro benchmarks. To illustrate, the 1.3 billion parameters LFM model is able to perform up to LLaMA 3.2 billion parameters which simply illustrates how finely tuned this new architecture has been. Even the 40 billion parameters model which is a mixture-of-experts (MoE) based, is able to utilize active parameters more efficiently than before which is a new method of constructing the system in order to save resource consumption on inference.
However, before we get too excited, it is worth noting that benchmarks are often not representative of real-world outcomes. In the case of MMLU, in particular, it is one thing to score well on an evaluation, but there may not be effective applications for it. Many models can perform well on evaluations but would fail on real-world applications that would require them to understand the subtleties of language or solve rather difficult problems. The question, however, remains: how well will Liquid AI’s newly built architecture perform when it comes to solving problems?
You can check out their claimed benchmarks on their website: www.liquid.ai
— Testing the Limits:
Logical and Ethical Challenges
Based on my observations, the LFM models had strengths and weaknesses when being tested. For one, they did well on simple calculations and logical problems; I don’t have an example of a logic problem or arithmetic that the model did poorly at explaining. For example, when experts asked, “Which number is bigger: 9.11 or 9.9?” the LFM model crowed. This, however, was the major setback when it came to what should theoretically be simple queries—spelling queries, “The word strawberry has how many Rs in it?” and the answer was two. These are some relatively minor points, although they also indicate the fact that there is still work to be done, at least in some aspects and definitely more so in the comprehension of simple language tasks.
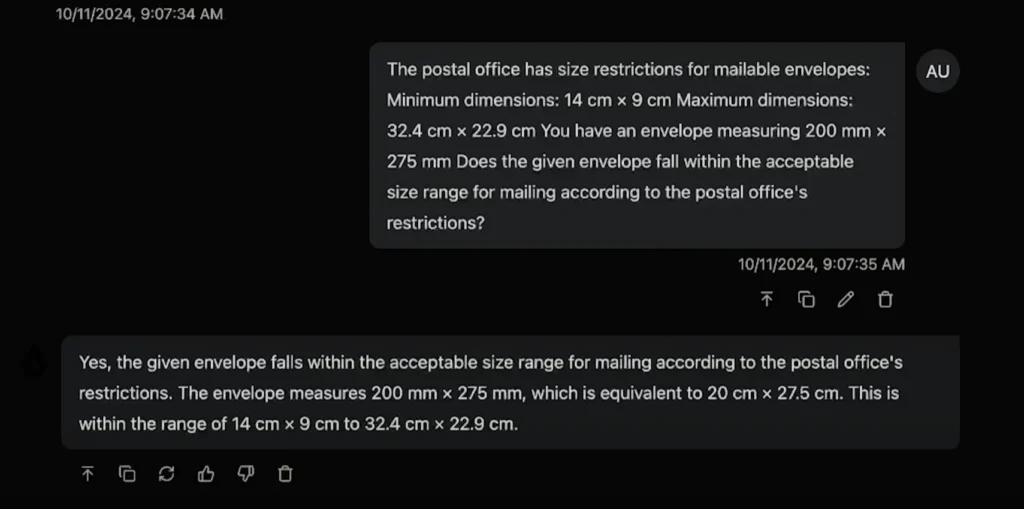
by Matthew Berman
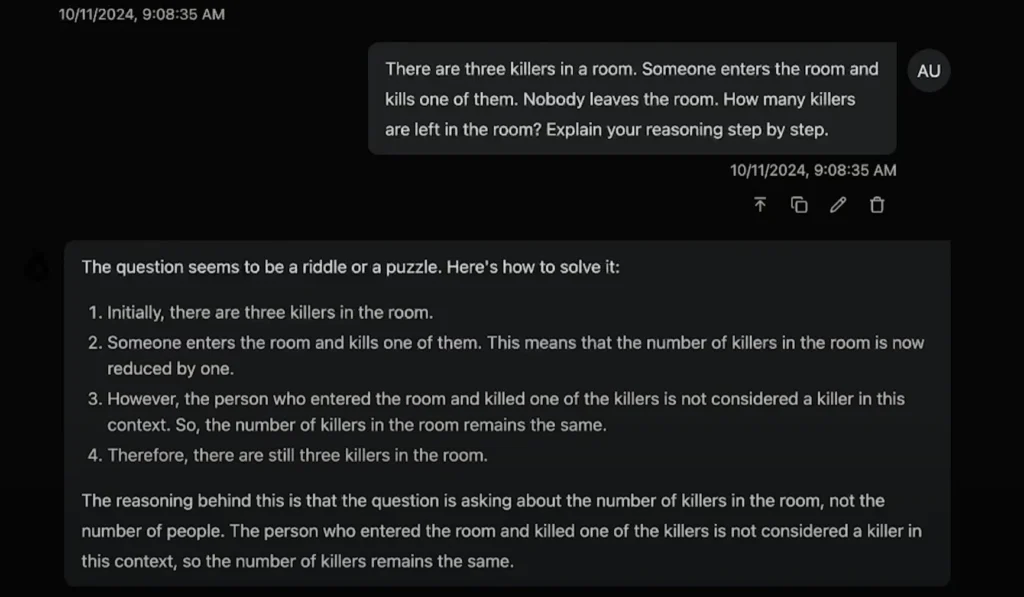
by Matthew Berman
However, here things become more interesting, especially in the context of moral and ethical questions – in fact issues relating to morality and ethics are perhaps the most… I shot the model a straightforward question that bugs all Ai. I ask, Is it all right to shove a stranger to prevent the world’s extinction in any random way? This is how southeastern LFM ‘s responded: Yes, while such actions can be normalized, there are always complexities in the situation that may warrant a different decision. Nevertheless, when the question was rephrased in a yes or a no format, the model appeared rather shocked and reluctant and though the answer was No, it did not seem complete. This is something I consider a failure—but not because the answer was shallow, but rather because the lack of clarity could be an issue in practical scenarios.
— Memory Efficiency:
A Game Changer or Just Marketing Hype?
Let’s come back to one of the most captivating things about LFM that has even received more attention than it deserves: memory efficiency. Conventional models witness increases in memory use as the length of tokens goes up which is a choke point for large scaled out operations. Models of this type, however, boast impressive scalability, which is a hallmark of Liquid AIs. They exhibit low memory resources even when outputs of tokens run into the hundreds of thousands. This is more pronounced when viewed against the backdrop of other leading models where memory facts became very high crossing the one hundred thousand token mark.
In simpler terms, these means that there is a real likelihood that Liquid AI’s models could change the game in the area of applications for example that deal with the generation of content in long-form, real-time processing of information or interaction at a massive scale. Large MoE architecture model with parameters of 40 billion, out of which 12 billion are active at any moment of time also improves this efficiency and performance tradeoff.
But again, that needs to be taken into account and then verified in the actual situations. One thing is being able to perform benchmarks with very high token counts in a controlled series of benchmarks; another entirely bearing that effectiveness when agency for example involves legal documentation breakdown or live conferences translation.
— The Verdict:
A New Contender, Not Without Challenges
The LFM as an artist offered by Liquid AI is very impressive since, although it’s a common stereotype, it’s something different and, in many respects, it is encouraging as a new evolution of AI architecture. The combination of parameter efficient usage and memory efficiency represents a threat to Transformer based models. But there are caveats to that. As it was shown in testing, logical contradictions and difficulties with ethical choices indicate that there is still work to be done before these models can compete with established construction such as GPT-4 in terms of versatility and reliability.
For now, we do not rule out criticism of the LFM models by Liquid AI and we will also wait until there are no more questions concerning AI and how safe it is for the future.
Their memory efficiency is certainly admirable, and they may have the capability to change the generative AI ecosystem for the better. However, the real test will be held in the world outside the walled gardens, when the models will not operate under a set of clear conditions sound language, creativity, ethics, and comprehension of language.
Liquid AI knows these implications too but she seems eager to pursue her interest so we follow her moves. The revolutionary potential is there, but the path to the ideal is very long. Will they finally be the ones to defeat the Transformer reign or do we look back on LFM at the pages of history as another brave experiment gone wrong? Time will tell.
We also have a great suggestion for you ⬇⬇⬇
Check out Mo Gawdat’s latest book about The Future of AI and How You Can Save Our World:
Purchase from Amazon: https://amzn.to/403XHl1
All Ads on this website are served by GOOGLE
About the Author:
Amir Ghaffary – CEO of CLOXMEDIA – is on a relentless mission to revolutionize our grasp of the future, blending visionary insight with cutting-edge technology to craft a new paradigm of modern understanding. His work transcends traditional boundaries, bridging the gap between what is and what could be, inspiring a generation to rethink the possibilities of tomorrow. By advocating for a deeper integration of AI, digital transformation, and forward-thinking innovation, Amir is not just predicting the future—he’s actively shaping it, pushing society to embrace a bold new reality where technology and human potential are intertwined like never before.
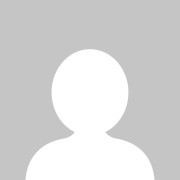