Through an examination of key breakthroughs—such as large-scale pre-training, test-time (inference) compute, and reinforcement learning with verifiable rewards—this article delineates how AI systems are rapidly moving towards novel scientific discovery. With exponential growth outpacing Moore’s Law, AI now appears poised to alter the very fabric of society by revolutionizing computer programming, medical research, and other knowledge-intensive domains. This research was conducted by the CLOXLABS team, for the purpose of informing the readership of CLOXMAGAZINE about these transformative trends.
◗ NOTE: This is an official Research Paper by “CLOXLABS“
Introduction
Since the dawn of digital computing, scholars and engineers alike have pursued ways to amplify human cognitive tasks. Modern AI systems, from generative text models to game-playing programs, have demonstrated profound progress, sometimes at a rate exceeding most expert projections. According to Sam Altman—one of the leading figures in AI innovation—this progress is advancing in ways that suggest we may soon see AI agents capable of surpassing humans in tasks requiring high-level reasoning.
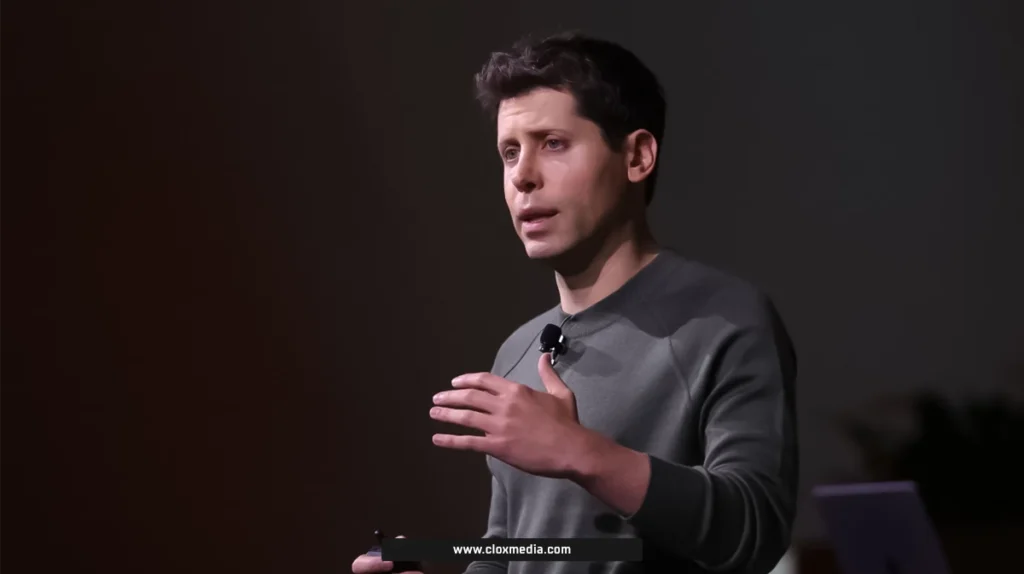
In particular, Sam Altman’s comments made in Japan and subsequent official blog posts strongly underscore how test-time compute (i.e., the amount of computational reasoning an AI model can perform after being trained) has empowered AI to improve in leaps and bounds. Traditionally, significant leaps in AI capabilities occurred via large-scale pre-training alone. Now, however, test-time compute offers an entirely new avenue for AI to iterate and refine its reasoning.
Altman has highlighted how prior generative language models (GPT-1, GPT-2, GPT-3, GPT-4, etc.) benefited from incremental scaling by factors of approximately 100×. Each increment not only broadened the model’s raw knowledge but also led to surprising, “emergent” features in language understanding. The addition of test-time compute, exemplified by models designated “01,” “03,” and specialized derivatives such as Deep Seek, magnifies these gains, enabling AI to process and verify solutions in real time. The critical advantage of these advanced techniques lies in their capacity to harness reinforcement learning with verifiable rewards.
Emergence of Superhuman Coding and Reinforcement Learning
One of the most tangible achievements on the AI horizon lies in superhuman coding. Models can now train on vast repositories of programming data and verify solutions through iterative processes where correctness is explicitly quantifiable. For example, a correct program passes a set of coding tests or solves a well-defined puzzle, and if the AI’s output meets these criteria, it receives a “reward.” Over repeated cycles, the system refines itself until it outperforms typical human benchmarks.
In contexts such as mathematics, reinforcement learning can also be highly efficient because solutions are unequivocally right or wrong. This precision fosters rapid model improvement. However, when it comes to creative tasks like poetry, where correctness is subjective, reinforcement learning becomes more challenging to formalize.
Although some experts were taken aback by Deep Seek’s capabilities, veteran AI researchers like Sam Altman and Dario Amodei foresaw how dropping compute costs and continuous model optimization would accelerate breakthroughs. As Altman has detailed, coding tasks in particular benefit from this synergy of enormous datasets, strategic model architectures, and verifiable reinforcement signals—leading to exceptionally refined AI outputs. Indeed, the efficiency gains in coding models have been so dramatic that Sam Altman predicts AI-based coding agents will surpass every human coder in the world by late 2025, effectively opening the door for continuous self-improvement loops.
Exceeding Moore’s Law
Moore’s Law, describing the doubling of transistor density roughly every 18 months, served as the central paradigm of computational progress for decades. However, according to Sam Altman’s newest blog post, AI is exceeding those predictions by an even more aggressive margin. In practical terms, the cost of inference—i.e., running queries on a large language model—has been dropping at a rate of around 10× every 12 months. This cost reduction, which is significantly faster than the historic rate of transistor scaling, makes advanced AI increasingly accessible.
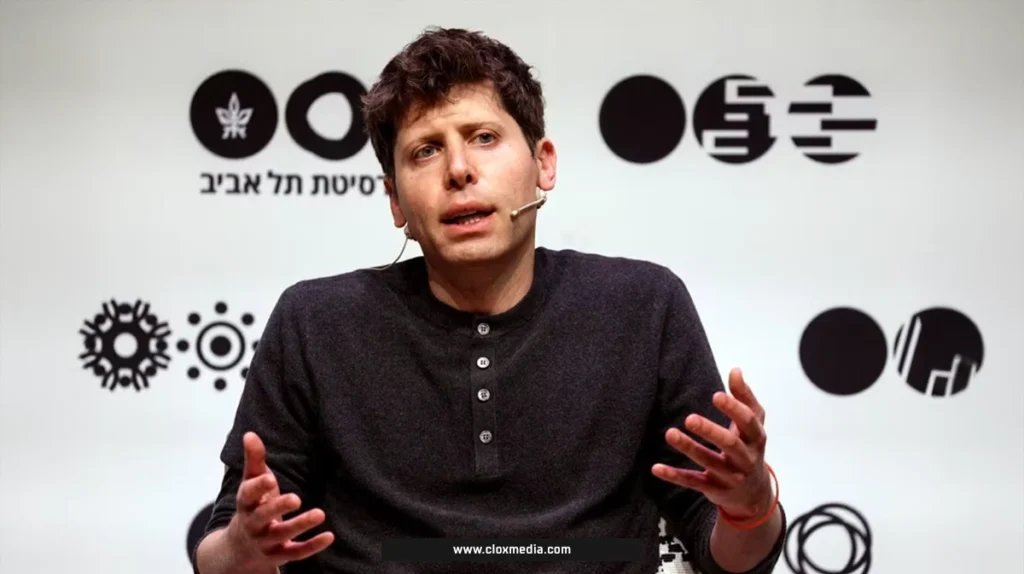
Accordingly, each time the cost to deploy a certain level of AI intelligence is slashed by an order of magnitude, more individuals, corporations, and research labs can afford to integrate AI into their workflows. This stimulates yet more data collection, more feedback signals, and ironically more investment in compute, fueling a self-reinforcing feedback loop.
Path to New Knowledge Discovery
One of the most transformative possibilities on the near horizon is the application of advanced AI to novel scientific discovery. Sam Altman underscores that current state-of-the-art models (like GPT-4 or variations with extensive test-time reasoning) are already adept at coding, mathematics, and structured problem-solving. In the next two scaling leaps, we could see systems that reliably propose or verify new hypotheses—marking the moment where AI becomes a fully fledged researcher.
The intelligence explosion scenario proposed by Leopold Aschenbrenner suggests that once models become able to generate new scientific understanding (and can apply these insights to improve themselves), humanity could experience a phase of unprecedented growth in knowledge and productivity—often referred to as the “intelligence explosion.”
All Ads on this website are served by GOOGLE
The Human Workforce in Transition
Coding stands to be the first major knowledge-based sector to be radically transformed. AI agents specialized in code generation will be endlessly replicable, never tire, and can operate at extremely high speed. This new cadre of AI “developers” could, in principle, tackle software engineering tasks around the clock, limited only by the available computational resources.
As has been seen with other game-changing AI demonstrations (e.g., AlphaGo surpassing top-tier human players in Go), the logic remains consistent: once an AI system outperforms human champions, the technology scales quickly to many domains. Similarly, a top coder model—replicated at scale—could engage with large-scale software development, dramatically lowering time-to-deployment and accelerating software innovation. Though this shift will inevitably raise questions about how to integrate AI developers alongside human coders, it also promises to free human programmers from rote tasks, providing more time for creative, strategic, and ethical considerations.
In parallel, AI agent workforce integration is expected across fields beyond coding. Accounting, legal research, clinical diagnostics, architectural design—any domain that requires methodical, knowledge-intensive tasks—may leverage these advanced AI capabilities. The net effect on global productivity will be immense, especially as cost reductions make these tools universally accessible.
Practical Examples and Sponsor’s Note
Polo AI is a shining example of how AI advances are not limited to text-based or coding tasks. Polo AI enables cutting-edge video generation by integrating multiple specialized open-source models into a single interface. The platform’s ability to do text-to-video, image-to-video, and video-to-video conversions mirrors the same shift we see in coding—a domain-specific capability, amplified by large-scale compute and verifiable metrics (in this case, style or consistency). With functionalities such as consistent character generation and seamless integration with advanced models like Cing, Runway, and Luma, Polo AI demonstrates how quickly creative tasks can shift from human-only to AI-assisted endeavors.
By deploying multiple AI video frameworks, Polo AI serves distinct uses—marketing, visual brainstorming, artistic exploration—and stands as an example of how cost accessibility combined with multi-model synergy can unlock novel possibilities. The overarching theme here is identical: the synergy of advanced architectures, test-time reasoning, and dropping costs are the catalysts propelling AI from impressive prototypes to ubiquitous, game-changing tools.
The AI sector today is in the midst of remarkable developments that point toward extremely capable, if not near-superhuman, performance in coding and potentially many other fields. Through breakthroughs in large-scale model pre-training, test-time compute, and reinforcement learning, these new systems are scaling in power at an astonishing rate.
Sam Altman’s acknowledgment that we may see the top AI coder surpass every human coder by year’s end highlights how close we are to an AI-driven era of research and productivity. The ongoing cost reductions—beating even Moore’s Law—ensure that these powerful AI capabilities will diffuse throughout industries rapidly. Indeed, as soon as AI becomes not only a tool but also a discoverer of new knowledge, humanity will enter a phase characterized by exponential growth in technology, scientific understanding, and overall societal impact.
About CLOXLABS: This research was spearheaded by the CLOXLABS team, a group of passionate innovators dedicated to exploring the frontiers of AI, society, and technological progress. We are proud to share these findings with the readers of CLOXMAGAZINE as part of our mission to illuminate the cutting-edge of AI and its broader implications for humankind.
All Ads on this website are served by GOOGLE